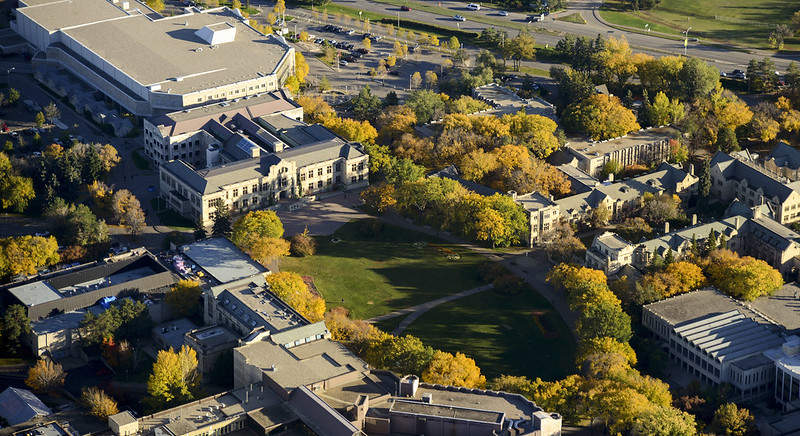
Math & Stats Colloquium – Friday, Sept. 23 @ 3:30 PM
Math & Stats Colloquium – Fri, Sept. 23 @ 3:30 PM in THORV 159 (Zoom link available)
The Colloquium Committee cordially invites you to attend the first talk in the 2022-23 Math & Stats Colloquium series, which will take place as a hybrid talk with the possibility to attend either in-person or in Zoom. We encourage everyone consider attending in person if possible.
Date/Time: Friday, September 23 @ 3:30 PM CST
Location: THORV 159 (please note the new location!)
Zoom Link: Please email colloquium@math.usask.ca for the link
Speaker: Csaba Szepesvari, University of Alberta and DeepMind
Title: A glimpse at reinforcement learning through the eyes of a theoretician
Abstract: Reinforcement learning (RL) is at the heart of a number of exciting recent developments in artificial intelligence. In 2013, RL was used to create a computer program that was shown to be able to play about 60 computer video games developed for a game console from the 1980s, achieving better scores than an average human does, while using the same inputs and controls than humans use. Shortly after, RL was also used to create a computer program that decisively defeated the best human player at the game of Go. In both cases, experts thought that creating these programs will take much more time and were especially skeptical of the value of an RL based approach to tackle these problems. After these successes, similar successes have been achieved in numerous other domains. Yet, RL has been around since for a long time. The basic ideas behind RL go back to the work of Richard Bellman and his coworkers, who worked on the fundamental problem that RL algorithms tackle in the 1960s. Of course, over the decades since then, computers got many orders of magnitudes faster, while recent years have also witnessed an even faster increase of investments into using computers (and in particular RL) to solve various problems. In this talk, I will explain the basic problem that RL tackles, the basic ideas that power today's RL algorithms, while I will also explain the foundational theoretical challenges behind all this. I will then connect the dots by speculating a little on how the foundational barriers were overcome in the previously mentioned examples and then close with a few open questions that would be important to address.
Please contact colloquium@math.usask.ca for the Zoom link or if you have any questions.
We hope to see you all on the 23rd!