The data gap
All scientific research depends upon reliable data, but it can be difficult to obtain and is often incomplete or flawed. Juxin Liu uses statistical tools to help health science researchers account for and analyze imperfect data.
WE’VE ALL LIKELY done it—enhanced the truth when asked about our weight, exercise or drinking habits. When trying to obtain accurate information on public health risks, however, these fibs can adversely affect the outcome of a study. For example, smoking while pregnant is underreported on health surveys, despite the increased risks of premature delivery and low birthrates that can lead to infant mortality.
Statisticians understand the world in terms of data: information gained by measuring or observing variables. The smoking status of pregnant women, long-term nutrition intake and physical activity are examples of variables that are difficult or costly to observe. Other variables in health science research are impossible to observe, such as depression or quality of life.
“These are more complicated problems,” says Professor Juxin Liu of the Department of Mathematics & Statistics. “Conceptually you can define these things, but how do you quantify them?”
Unveiling the “truth” masked by imperfect data is a vital area of research for statisticians like Liu. Breast cancer is the most common cancer in women worldwide, and although early screening programs have reduced deaths from the disease, proper diagnosis—particularly of hormone receptor (HR) status—is crucial to effective treatment. For example, post-surgery drugs that are highly effective in estrogen-receptor positive tumours are not nearly as effective in tumours that are estrogen-receptor negative.
But HR status is difficult to measure. It is also costly and depends on multiple factors, including specimen handling, tissue fixation, antibody type, staining and scoring systems—all of which are subject to error. Liu was lead author on a study of HR misclassification errors, working with her former PhD supervisor at UBC and clinicians from the University of Chicago.
Until 2010, the guidelines used by clinicians called for a tumour to be diagnosed with a positive HR status if 10 per cent of the sampled cells tested positive. Since then, the cut-off has been reduced to one per cent. Intuitively, one would think this a good thing, because the change increases the test’s sensitivity—the chance of correctly identifying HR positives. However, reducing the cut-off also decreases the specificity, meaning there is a greater chance of falsely identifying negatives as positives. The Bayesian methodology proposed by Liu and her colleagues takes this “tug-of-war” relationship between sensitivity and specificity into account in adjusting for misclassification errors.
Bayesian tools use historical information, or prior knowledge, in addition to the empirical data being analyzed. In the breast cancer study, the professional knowledge of clinicians was combined with the cut-off change to create a more accurate statistical analysis. It is one of the ways statisticians such as Liu assist other researchers in analyzing data that are misclassified, unknown or incomplete.
“For this study, the prior information about sensitivity and specificity comes from the clinicians’ expertise,” says Liu.
It’s her job to fill in the gaps.
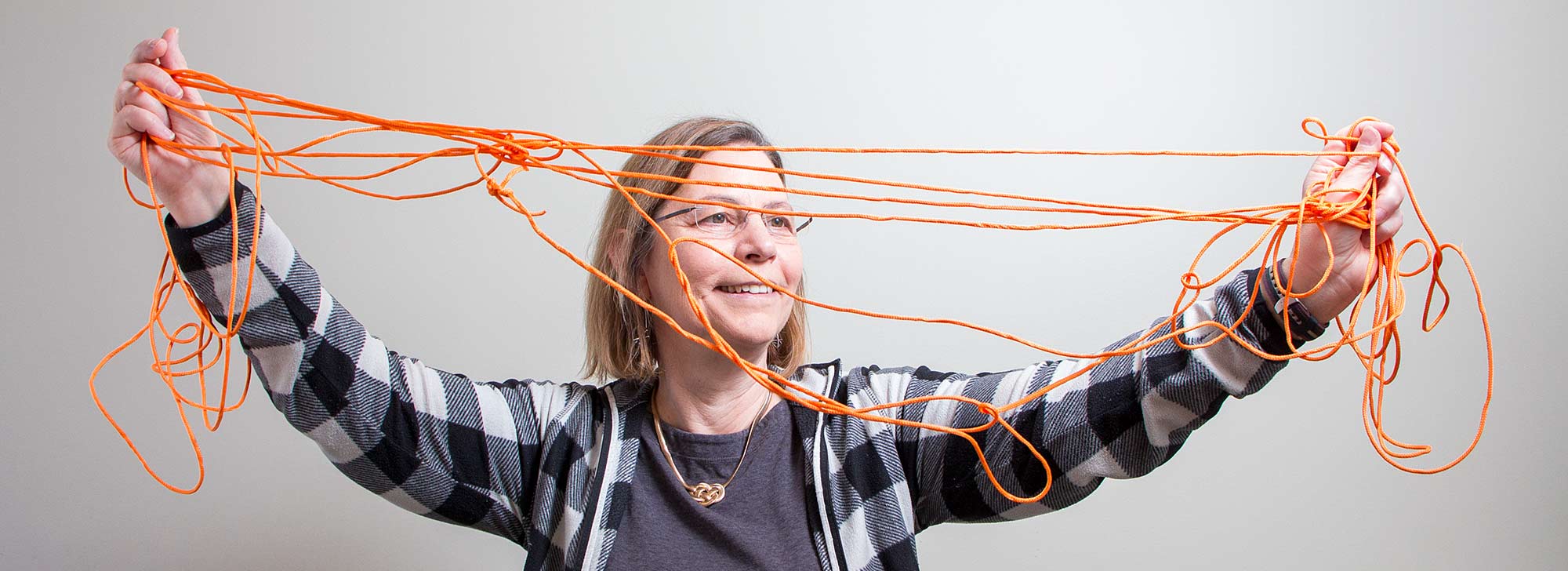
A tango with tangled polymers
WHILE STATISTICIANS ARE driven by real-world problems, mathematics professor Chris Soteros is motivated by the more esoteric behaviour of long-chain molecules, such as polymers and DNA, and the mathematical problems they pose. Her work involves analyzing the folding and “packaging” behaviour of these molecules. Given that two metres of DNA are folded into each cell in our body, studying the behaviour is daunting indeed.
To help unpack the problem, Soteros simplifies and simulates these molecules on a three-dimensional lattice, then uses mathematical tools such as random and self-avoiding walks to model their behaviour.
The sporadic path of a random walk is often described as “a drunkard’s walk home,” and is used to model random movements in large data sets—from stock market fluctuations to particle physics. A self-avoiding walk is a random walk that cannot cross the same path or retrace steps. Since no two atoms can occupy the same space, in three dimensions it is an ideal tool to model polymer behaviour.
To study polymer behaviour, Soteros models a polymer solution by using a lattice walk to represent the polymer and the empty spaces surrounding it to represent the solvent molecules of the solution.
In experimental solution at high temperatures, the polymer behaves like a self-avoiding walk. “At these temperatures, the polymer prefers to be close to the solvent molecules, but if you decrease the temperature, the polymer prefers to be closer to itself,” explains Soteros.
Surprisingly, at a specific lower temperature the polymer behaves like a random walk, and below that temperature a “collapse” transition occurs, and the polymer folds in on itself.
“It wasn’t until the late ‘70s that the collapse transition was observed in the lab, and you had to have a very large molecule in a very dilute solution to see the transition,” says Soteros. “This is an example of mathematics predicting a behaviour before it was confirmed by experiments.”
Sometimes theories are discovered the other way around. Former student Michael Szafron (MSc’00, BEd’09, PhD’09)—now assistant professor in the School of Public Health—came to Soteros with a complex problem. Long strands of DNA can become knotted when packed into the confines of a cell nucleus, but in order to replicate successfully, DNA must be unknotted. Enzymes called type II topoisomerases perform the necessary untangling by cutting one strand of DNA, passing the other strand through the break and then reattaching the ends of the broken strand. How does this startling solution work so well, and how can it be mathematically modelled?
It helps to imagine a long necklace that has a knot; unfastening the clasp helps to untangle the knot. “The problem is that a necklace clasp could be far away from where the knot is, so it would be difficult to pull it through,” says Soteros. Yet these enzymes seem to know exactly where to cut the DNA.
By modelling the basic behaviour of very large molecules in solution, Soteros is building mathematical evidence to understand how these enzymes work so efficiently—and how they might be used to develop new antibiotics and anticancer drugs.
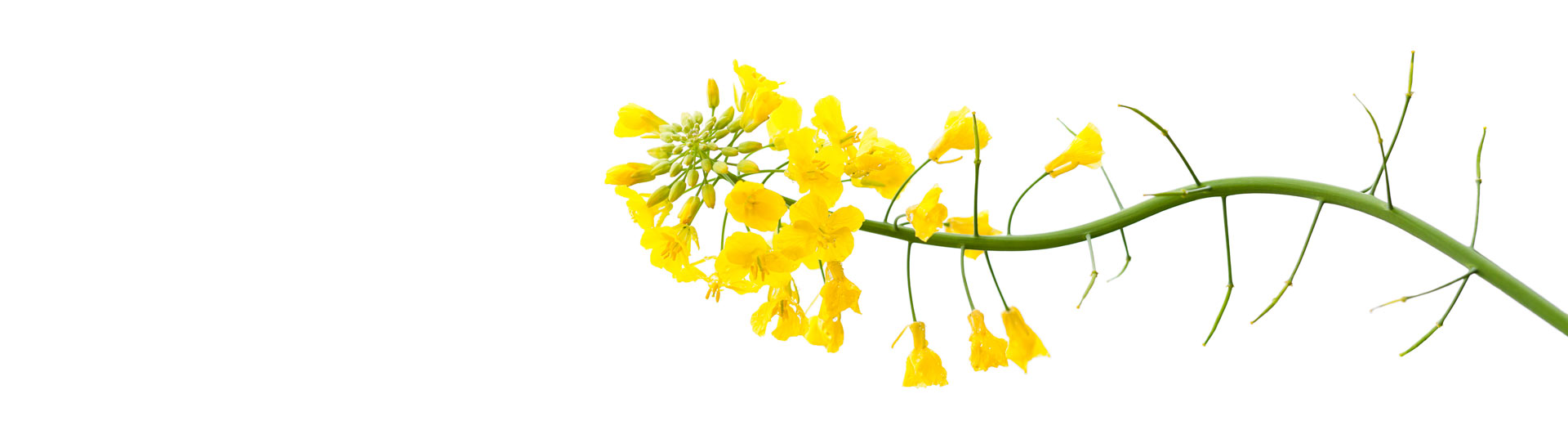
Rendering fat data with self-learning algorithms
BIG DATA COME in various shapes. Transactional data mined by businesses such as Facebook or Superstore typically have many subjects (us) and few features. Scientific data most often have fewer subjects and many features (a genotype). If you consider data from a two-dimensional viewpoint, the first type is tall and the second is wide. Statistics professor Longhai Li uses machine learning to cut through the fat on large, wide datasets.
As a statistician participating in a Canada First Research Excellence Fund (CFREF) project, “Designing Crops for Global Food Security,” Li has been studying the effect of plant genotype on phenotype, or the physical characteristics such as leaf shape, plant height and water absorption—all factors that affect yield.
Li has also been investigating how the abundance of certain bacteria might influence phenotype as well, and could ultimately affect crop yield. “This is what many scientists suspect, so we want to discover what bacteria affect which phenotype,” Li says.
The number of features in a genotype plus the number of possible bacteria result in two very fat datasets. Li uses machine learning—the intersection of statistics and computer science—to tackle the intractable problems posed by big data. Li received funding from the Canada Foundation for Innovation (CFI) to establish his own computer cluster of 300 computer cores to deliver the enormous computing power required.
“It’s an essential tool for all of my research and collaborations,” he says.